Gender bias in AI and ML – balancing the narrative
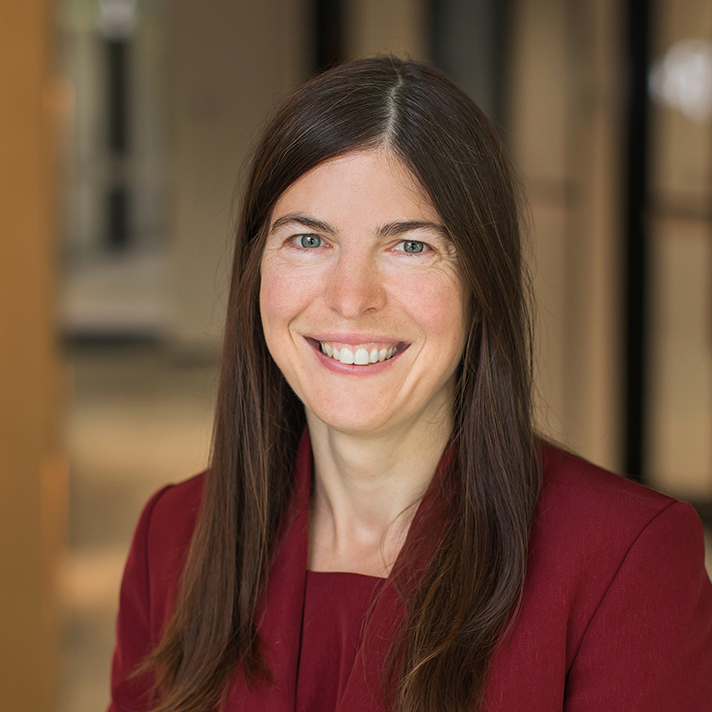
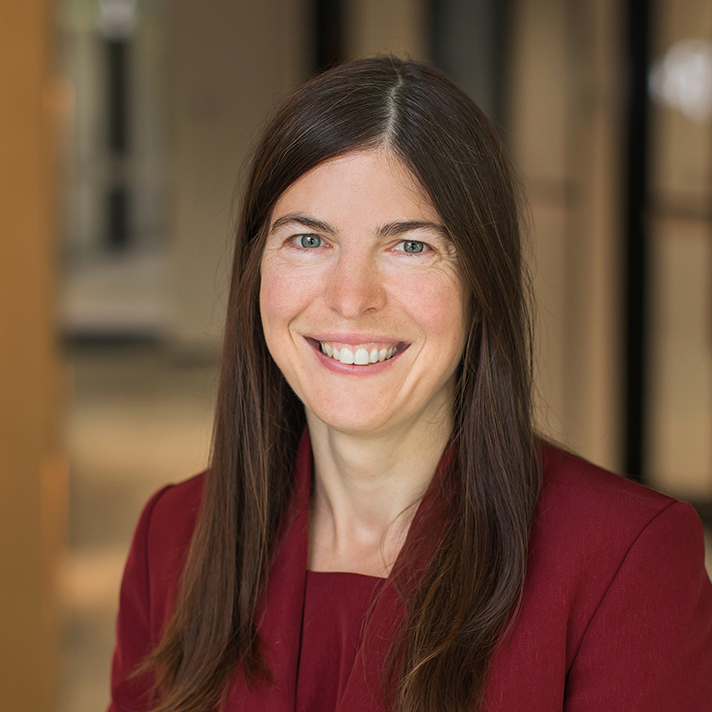
In support of this year’s International Women's Day theme Cracking the Code: Innovation for a gender equal future, statistics expert and Taylor Fry Director Gráinne McGuire looks at the issues impacting gender parity in this age of digital transformation. Driven by her passion and advocacy towards the ethical use of machine learning (ML), Gráinne explores the inherent gender bias in artificial intelligence (AI) and what can be done to close the gender gap.
“Man is to computer programmer as woman is to homemaker?”. So opens a paper I came across recently. It seems to me to perfectly encapsulate the problems with gender and AI. Not that there’s anything wrong with either job – what’s wrong is the gendered association. Combine that with AI and ML being increasingly applied at scale and in a position to not only predict the future, but also create the future and reinforce biases, and we’re into Cathy O’Neil’s Weapons of Math Destruction territory.
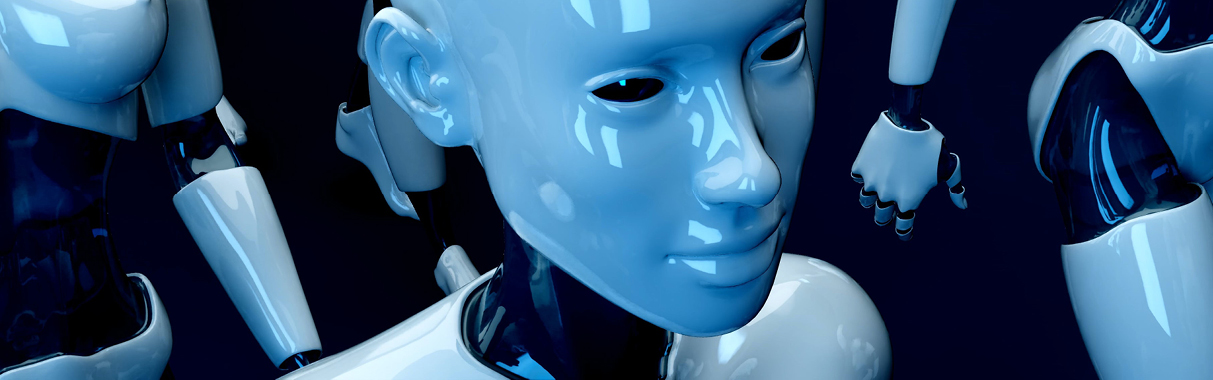
If data is biased, AI and ML tools amplify the biased patterns, such as woman = nurse
We know we’ve got problems with AI and gender. AI relies on lots and lots of data. This data comes from our lived experiences and our world is biased. And AI isn’t really all that smart – it just pretends to be by being really good at finding patterns in data and using those to infer or predict things about the world. And therein lies the problem – AI suffers from bias amplification. You feed in biased data and – no surprise – the AI or ML tool finds the patterns and comes up with man = doctor, woman = nurse. Or the one above, which isn’t necessarily all that accurate. Women were prominent in the early days of computer programming after all – with the likes of Ada Lovelace, often regarded as the first computer programmer, and American computer scientist and mathematician Grace Hopper paving the way – until men realised it was cool and important.
Those in the know might point out that I’m referring to embedding models above and in many ways they are the larvae to ChatGPT’s butterfly (related, but utterly different and so much upstaged by ChatGPT), but ChatGPT can fall prey to the same thing (although the ChatGPT creators do appear to have deeply considered gender bias – still, it’s hard to catch everything).
Real-life examples of gender bias in AI
Profession gendering
In a recent article for Fast Company, Textio cofounder Kieran Snyder asked ChatGPT to write feedback for someone in a particular job with no gender specified. Often the feedback was gender neutral but stick nurse or kindergarten teacher into the mix, or mechanic and construction worker and the she’s and he’s start to appear. You can probably guess which jobs got which pronouns. But at least doctors were always ‘they’ in their tests, so that’s something.
Racial bias – even famous people are not immune
Of course, it’s not just language models that suffer from bias and specifically bias against women. We see it in facial recognition software, particularly if you’re a black woman. MIT graduate, Joy Buolamwini’s research paper uncovered large gender and racial bias in automated facial analysis algorithms. Given the task of guessing the gender of a face, the systems performed substantially better on male faces than female faces, with error rates of no more than 1% for lighter-skinned men, compared with error rates of up to 34.7% for darker-skinned females. She called this phenomenon the ‘coded haze’, in which AI systems failed to correctly classify the faces even of iconic women like Oprah Winfrey, Michelle Obama and Serena Williams.
The’ tech bro’ age
And coming back to where we started, man is to computer programmer? Well, that’s a contributing factor to the problem. We’ve all seen pictures of the tech bros. Even those of us not in tech but using AI/ML tools in other areas often find ourselves in workplaces that have more men than women. And when people are designing algorithms and thinking about their impacts, with the best will in the world, it’s hard to be a good advocate for someone whose experience of life is very different to yours.
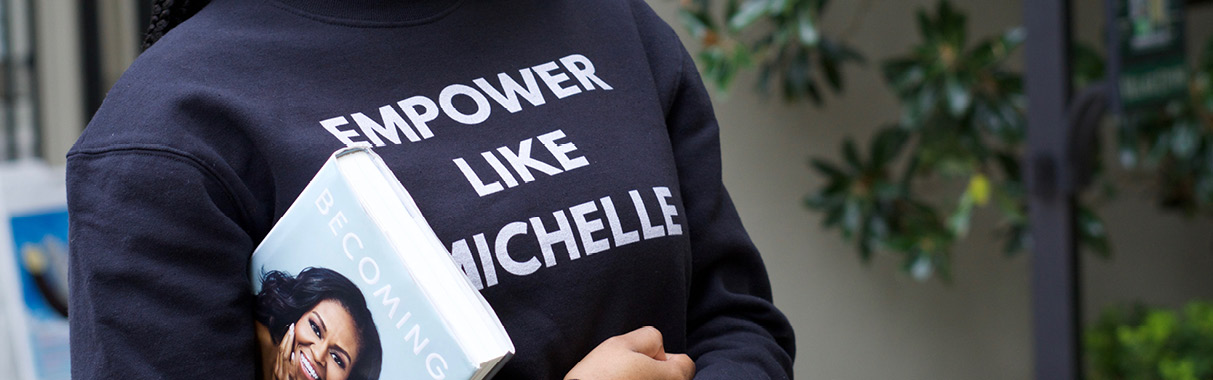
Bias in facial recognition has seen algorithms fail to classify iconic women like Michelle Obama
A matter of life and death – why we must act now
Quite literally, this bias is killing people. Medical data, I’m looking at you. A lot of medical trials are carried out on men only because – you know – women have these awkward hormonal cycles that mess with the results. So, let’s do it on men only and get nice clean results. But, those very same hormones might mean the medicine works differently in women, or their symptoms might be different. Feed that inadequate data into an ML algorithm and the bias goes round and round. Women get inadequate health care or die.
Invisible women – when prejudice is magnified
This problem was explored in depth by best-selling author Caroline Criado Perez, who spent years investigating the gender data gap, and wrote the award-winning book Invisible Women. In her new podcast series, Visible women, Caroline uncovered that AI might be making healthcare worse for women because it magnifies the pre-existing bias and data gap caused by overrepresentation of men in cardiovascular research. In fact, according to research funded by the British Heart Foundation, more than 8,000 women died between 2002 and 2013 in England and Wales because they did not receive the same standard of care as men.
The ‘strong’ vs ‘bossy’ lens
In a wide-ranging interview with Jacqueline Nolis, a data scientist, I was struck by Nolis’s experiences with performance reviews as a transgender woman before and after transitioning. A well-established data scientist, her reviews went from being described as being “so good at always saying the truth … even in hard situations” and “thank goodness … always speaking out” to – following a job change after her transition – receiving for the first time in her life reviews like “difficult to work with”, “doesn’t know how to speak to other people”, “needs to learn … when to not say stuff”. Is it any surprise we’re losing women from the AI/ML pipeline when many of them face everyday sexism?
Busting myths one hurdle at a time
And that’s even assuming they get into the pipeline in the first place. They have to get past the hurdle of studying a STEM field in university, where many women come from a society that says “women just aren’t as good at maths and sciences as men”. This even became a controversial political issue in England in 2022 when the Chair of the Social Mobility Commission discussed why fewer girls take A-level physics, saying “they don’t like it, there’s a lot of hard maths in there that I think they would rather not do”. While not everyone shares that view (the Children’s Commissioner for England countered that it was more to do with the lack of female role models in STEM), it does show that this view is endemic in many societies.
AI might be making healthcare worse for women … More than 8,000 women died between 2002 and 2013 in England and Wales because they did not receive the same standard of care as men.
What can be done to close the gap?
This is hard – let’s not minimise it. But hard is not an excuse for doing nothing.
Tackle ethics together
Ethical data standards are a place to start and there are many of these around, but they all (unsurprisingly) share common themes – recognise and manage bias, be fair, consult with those impacted by their use, consider privacy and human rights. However, it’s important to acknowledge the problem with many of these standards is that they don’t tell you how to deal with these issues.
This point was demonstrated in feedback we received in our one-year review of the NZ algorithm charter (a “commitment by government agencies to manage their use of algorithms in a fair, ethical and transparent way”). Some of the feedback included that not all agencies had sufficient experience in measuring bias or applying human oversight, and they’d appreciate a community of practice to support compliance with the Charter as a whole. So we need to learn from one another.
Focus on fairness
There’s much discussion around the concept of fairness out there. But fairness is a complicated problem, with many possible and conflicting definitions. I’ve discussed fairness in the past, so I won’t repeat it here (but if you check out the article, you’ll find an example of gender bias in Swedish snowploughing, as well as a discussion around some issues of fairness).
If you’re building a model with significant personal impacts, then you may want to consider building an interpretable model, as per another article of mine on the topic. Long story short, if you’re looking to deal with biases, then working with interpretable models can make this a lot easier, since you at least understand why your model makes the predictions that it does.
Proactive perspective in the workplace
Consider your staff and get a diverse range of people into the room working on these algorithms, which in turn gives you a diverse range of views on the possible consequences of using them. I’ve observed that the outspoken people on topics of fairness, bias and impact of AI are often women – Cathy O’Neil, Timnit Gebru, Cynthia Rudin, Caroline Criado Perez to name a few. Is this a coincidence?
The thing is, gender isn’t a minority group, women and men are approximately equal in number. And if AI is biasing against close to half the population, that’s a huge problem we need to solve.
Understand that self-promotion can be nuanced
I’m not an HR person so it’s a bit outside my area of expertise to suggest how to do this, but there are some commonsense things we can do – like recognising that, overall, women are more likely to underestimate their abilities, men to overestimate. Or that people may come from cultures where self-promotion may be frowned upon. When making hiring or promotion decisions, we should take that into account.
Encourage more women in STEM
Finally (in the sense of this article, not in the sense of solutions to the bias problem!), we’ve also got to get more women into the STEM pipeline – more girls doing STEM subjects at school and university. Again, this is a huge subject in its own right, so I won’t go into details here. But I will note that one of the articles I referenced earlier, discusses recent research by the authors which found that external feedback on mathematical abilities had a significant impact on the likelihood of girls pursuing a maths-requiring STEM degree.
Crucial flow-on effects of fixing gender bias
Let me finish by acknowledging that gender isn’t the only thing AI has a bias problem with. Personally, being a cisgender white female puts me in a far better place than many others find themselves. Minority groups in general suffer, some more than others. But we shouldn’t fall prey to whataboutism and use that as an excuse not to fix gender bias because other groups have it worse. What’s more, a large proportion of those other groups who have it worse will be women anyway, so fixing gender bias may just help them out a little bit, too.
The thing is, gender isn’t a minority group, women and men are approximately equal in number. And if AI is biasing against close to half the population, that’s a huge problem we need to solve.
Related articles
Related articles
More articles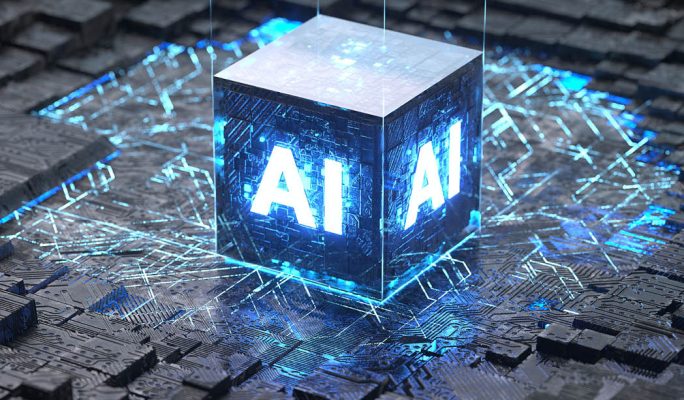
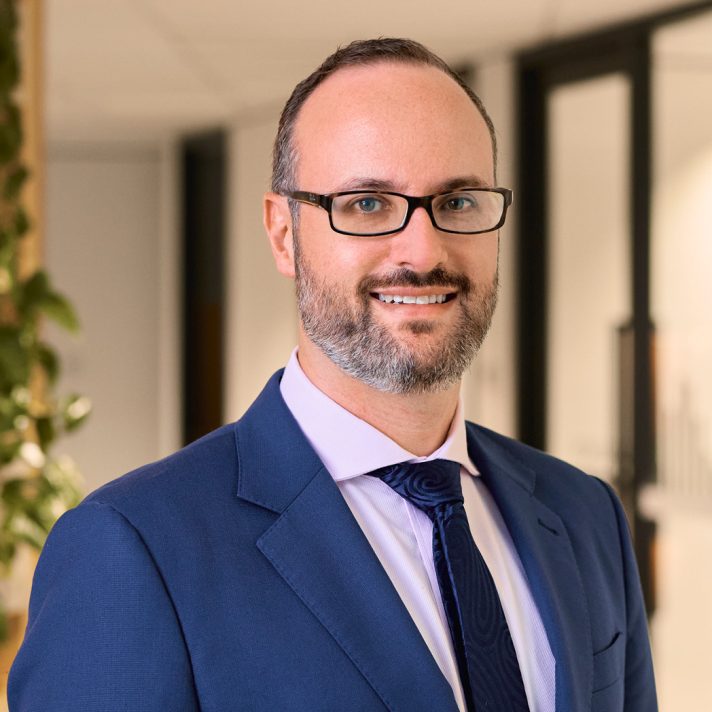
How AI is transforming insurance
We break down where AI is making a difference in insurance, all the biggest developments we’re seeing and what's next for insurers
Read Article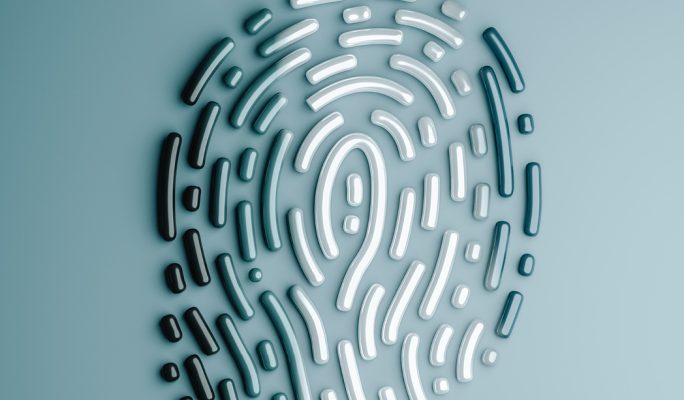
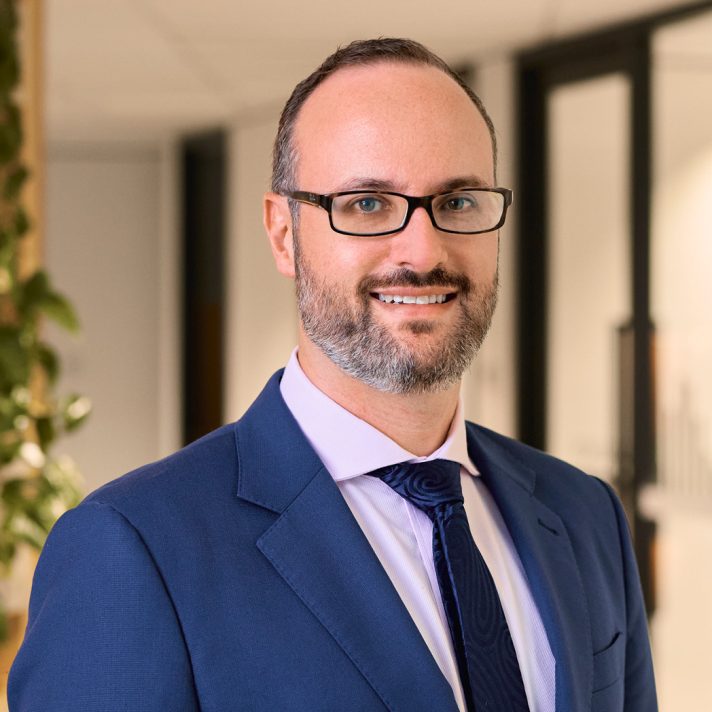
How AI will be impacted by the biggest overhaul of Australia’s privacy laws in decades
Understand the key changes to the Privacy Act 1988 that may impact AI and how organisations who use AI can prepare for these changes.
Read Article