Accuracy and causality the hot topics in data science at SIGKDD 2022
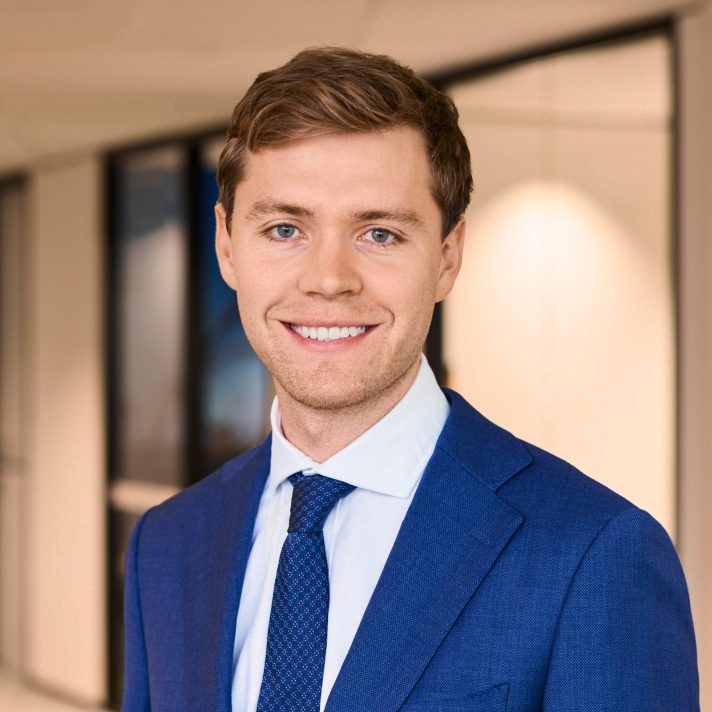
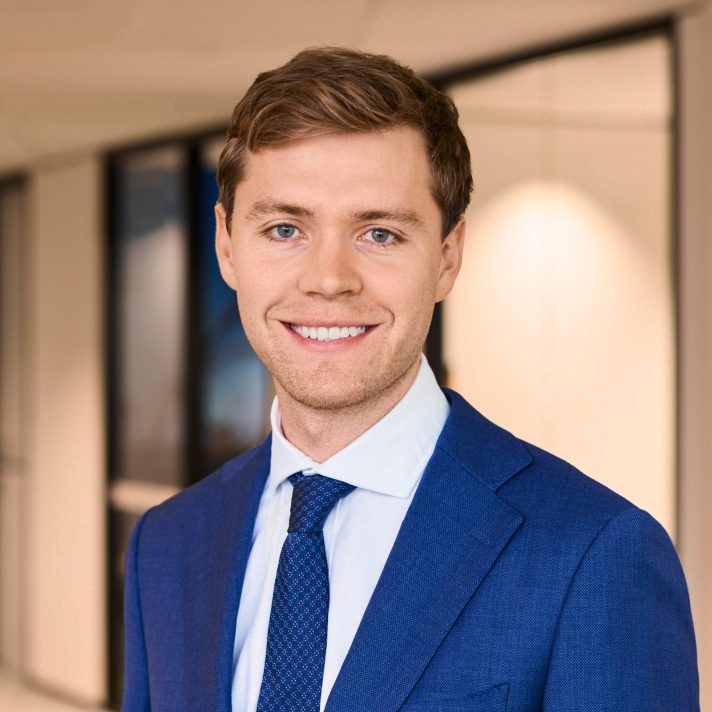
The international data science community gathered recently in Washington DC for the first in-person conference of its kind since 2019, sharing insights on knowledge discovery and data mining. Taylor Fry’s Tom Moulder was there for the 28th Association for Computing Machinery SIGKDD event, and reveals two big themes – why model accuracy is no longer enough and the growing embrace of causal relationships.
KDD styles itself as the world’s top interdisciplinary data science conference. It’s a fair assessment, given KDD has introduced some of the most influential developments in machine learning (ML) – for example, the now ubiquitous XGBoost algorithm.
This year featured speakers from Google, DeepMind, Amazon, DARPA, Microsoft, Meta, LinkedIn and the world’s top universities. Talks spanned a range of applications, including recommender systems, social networks, health, autonomous vehicles, finance, social impact and ecommerce.
Despite this breadth, there were a couple of key challenges that featured consistently:
- Beyond accuracy – How can we build ML applications that are not just accurate, but also fair, unbiased, robust, privacy preserving and transparent?
- Capturing causality – How can we build models to understand causal relationships, and empower us to analyse the best decisions to make, rather than just predict outcomes based on history?
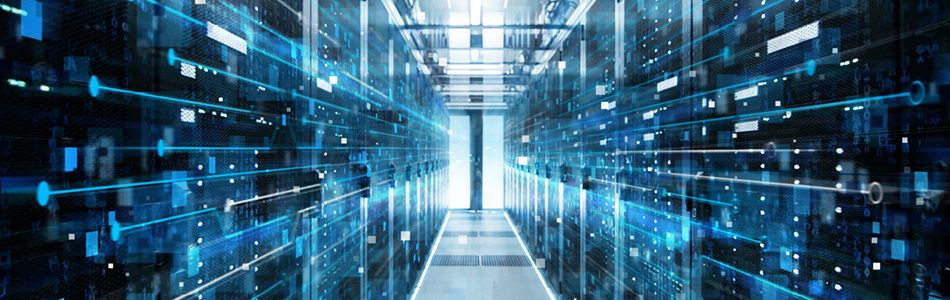
Causation analysis has relied on randomised experiments, often wildly expensive or impossible to run
Finding value beyond accuracy
As the ML field matures, models are increasingly required to be more than just accurate. They should also be transparent, explainable, fair, privacy preserving and robust to exploitation.
This is echoed in 2021 comments from European Commission Executive Vice President Margrethe Vestager, concerning European Union rules on artificial intelligence: “… trust is a must, not a nice-to-have”.
These challenges have been a focus of ML researchers and practitioners in recent years, appearing in KDD talks and workshops across almost every application and industry. The issues are particularly relevant for actuaries in our approaches to modelling and problem solving.
The argument for a human-centric approach
At the conference, Krishnaram Kenthapadi (Fiddler AI), Hima Lakkaraju (Harvard), Pradeep Natarajan (Amazon), and Mehrnoosh Sameki (Microsoft) presented a workshop on model monitoring, in a world where achieving model accuracy is no longer enough – rather, it’s just one of numerous considerations with potentially serious consequences. They make the case that continuous monitoring is critically important, and should involve evaluating biases, (un)fairness and model robustness rigorously. They argue for ‘human-centric’ modelling, whereby monitoring provides analyses to guide model review, while preserving human decision-making around questions such as when to review and update models.
Tackling bias
A full day workshop on Responsible Recommendations continued this theme, raising issues such as fairness in algorithms recommending job applicants for open positions. James Caverlee (Texas A&M) discussed the risk of ‘mainstream bias’, whereby recommendation engines may be biased towards performing well for mainstream users, but more poorly for niche users or minority groups. He went on to propose data augmentation and model tuning methods to tackle these biases.
Glassbox modelling – Powerful and explainable
Rich Caruana and Harsha Nori, of Microsoft Research, further emphasised the importance of metrics beyond pure accuracy, stressing model explainability and privacy in their new ‘glassbox’ modelling approach. This capitalises on the power of ML methods, while retaining a simple and explainable final model structure.
They demonstrated the advantages of their approach in a case study predicting risk of sepsis in pediatric hospital patients. Their explainable modelling structure highlighted the potential for patient risk scores to vary unexpectedly – likely due to data biases caused by treatment processes. Physicians were then able to manually edit the model to better reflect their knowledge and remove the unexpected effects.
The ability to understand and meaningfully engage with the model enabled physicians to improve and trust it – a crucial factor in applying models successfully.
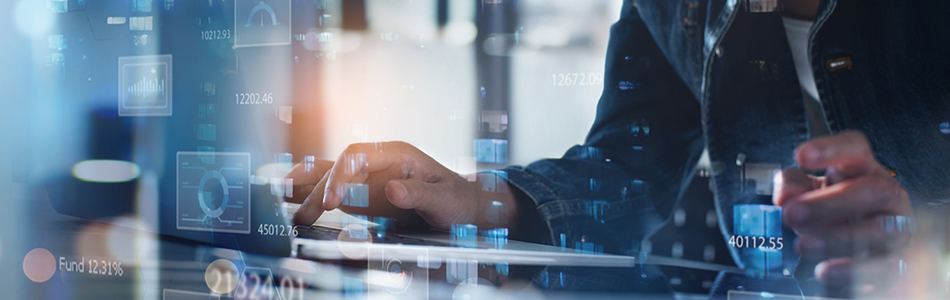
Applying models well requires understanding and meaningful engagement to improve and trust them
Correlation vs causation
Anyone who has spoken with a statistician will have heard the phrase ‘correlation does not equal causation’ ad nauseum. However, many of the highest value data science applications are questions of causation. Should I prescribe a patient medicine A or B? Charge price X or Y for my product? Invest in marketing campaign C or D?
Traditional analytics and ML methods aren’t designed with these questions in mind, and often fall short. They model correlations between variables, but don’t directly estimate causal relationships. Analysis of causation has relied on randomised experiments, which, while effective, are often prohibitively expensive or impossible to run.
Emphasis on causal relationships
In the past decade, developing methods to understand causal relationships has been a key focus of academia and industry. In 2018, world-renowned computer scientist Judea Pearl brought the idea of causality to a more general audience, with his widely popular The Book of Why. Most recently, the subject even featured in the 2021 Nobel Prize in Economics, which was awarded to Angrist and Imbens for their contributions to analysis of causal relationships.
In keeping with this trend, causal modelling was a key focus of KDD2022.
Making a difference for business
A team from Vaiani Systems discussed the benefit of causal approaches in business, using churn modelling as an example. They grouped data science tasks into three levels: Descriptive (what happened), Predictive (what will happen) and Prescriptive (what to do). While a predictive model can identify customers likely to churn, a prescriptive one distinguishes between those who will always churn (lost causes) vs those who can be influenced to stay (persuadables). Separating these groups allows marketing budgets to be focused on persuadable customers and improve overall retention.
They cite further examples from LinkedIn, Uber, Netflix and Amazon, where adopting causal approaches has made a real difference for the business and its customers. They also stressed the importance of developing offline evaluation and simulation infrastructure to support development and testing of causal analyses.
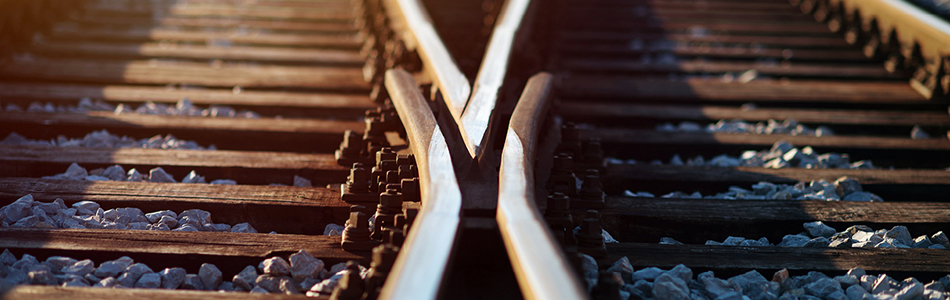
The best decisions don't necessarily come from models with high traditional predictive accuracy
What makes a good decision?
In public health, Professor Milind Tambe shared an example from his work as Director in at Google Research’s AI for Social Good. His team uses models to allocate resources to call centres, which provide health advice and reminders to pregnant women in India. While the team have seen good results so far, they have observed that models with high traditional predictive accuracy metrics do not necessarily result in better decisions in the field. He makes the case that this is because standard ML approaches focus on accuracy rather than decision quality. His team is now working on approaches to directly elevate decision quality – an approach conceptually aligned with causal modelling.
Improving evaluation tools
Tambe’s example is one of many where the standard model building and evaluation tools used by data scientists often don’t perform well on causal problems. The field is quickly developing new tools to fill this gap, with examples from this year’s KDD including new feature selection approaches for causal problems, and tutorials highlighting recent developments in multiple causal analysis packages.
This ties closely to the idea that models should be evaluated on more than just accuracy – the most accurate predictive model is not always the best one and does not always translate to high-quality decisions. In some cases, standard predictive models and evaluation metrics can be misleading to the point where more accurate models can result in worse decisions!
Where to from here?
Overall, KDD provides evidence of increasing recognition of the need for causal analysis, which improves decision-making across industries. While the range of tools available to meet this need is maturing, the problem remains challenging and relies on certain types of historical data collection. Where possible, experimentation is important to test whether predicted benefits are true in practice, and support future development of more causally focused models.
Ultimately, models should be evaluated with a holistic view of the problem they are trying to address. In practice, this means considering fairness, bias, privacy and robustness – beyond accuracy alone. Supported by interpretable model structures, this will help gain greater trust from end users and open the door to expert input on models.
Recent articles
Recent articles
More articles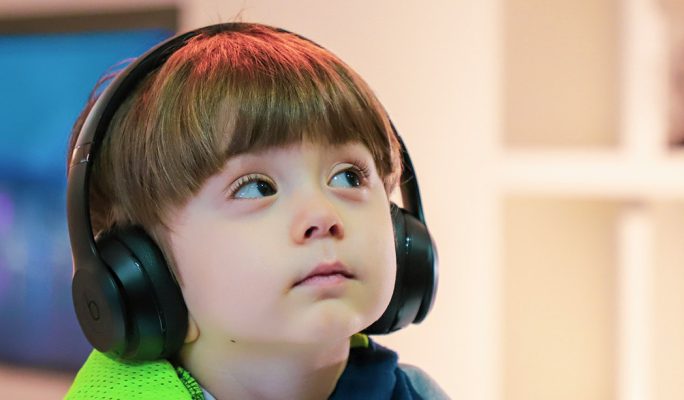
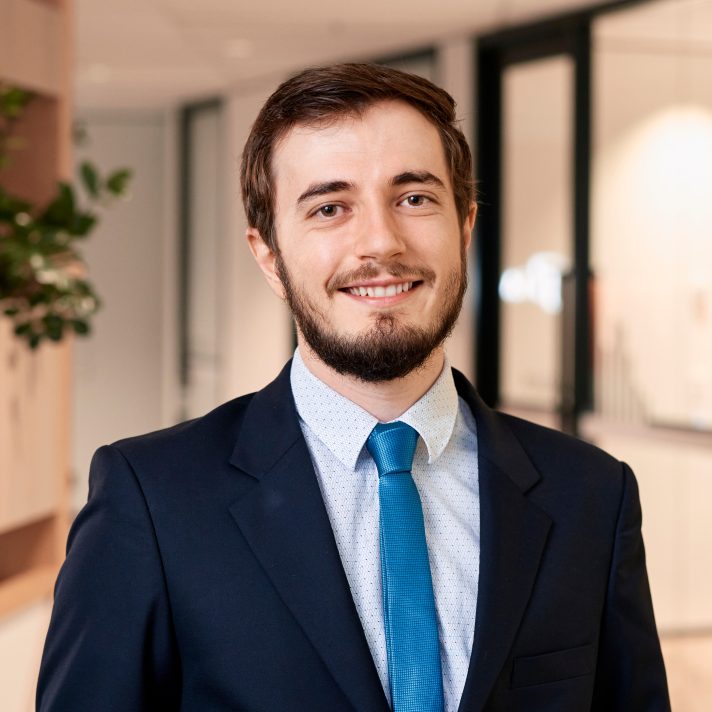
Increase in Australians with disability – new ABS survey
New data shows a significant rise in the number of people who report having a disability. We discuss the findings and offer key takeaways
Read Article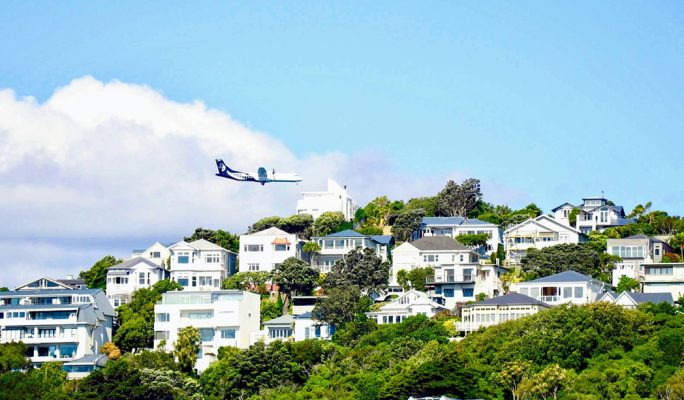
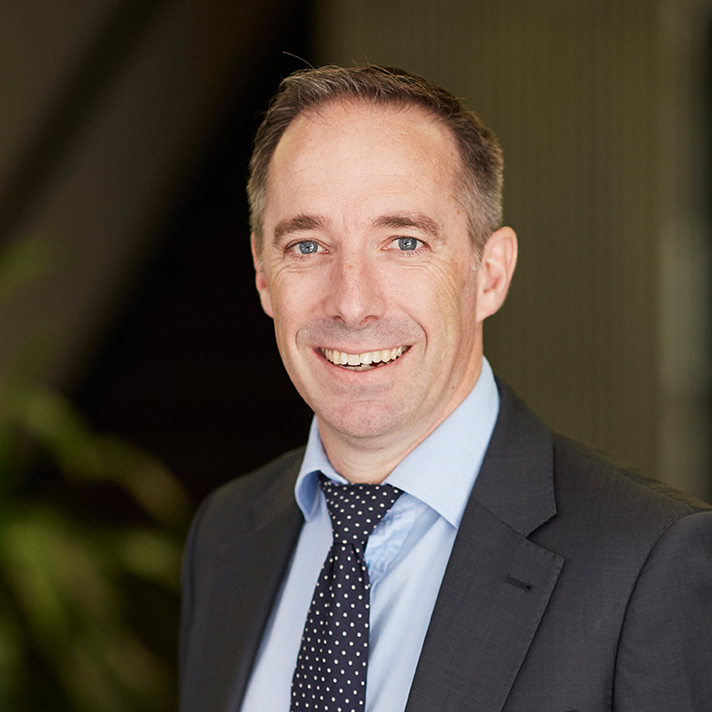
Elevated premiums and profits – what’s really going on in New Zealand?
A perfect storm may be brewing as Aotearoa community experience and insurer bottom lines collide. Ross Simmonds unpacks the issues
Read Article
Related articles
Related articles
More articles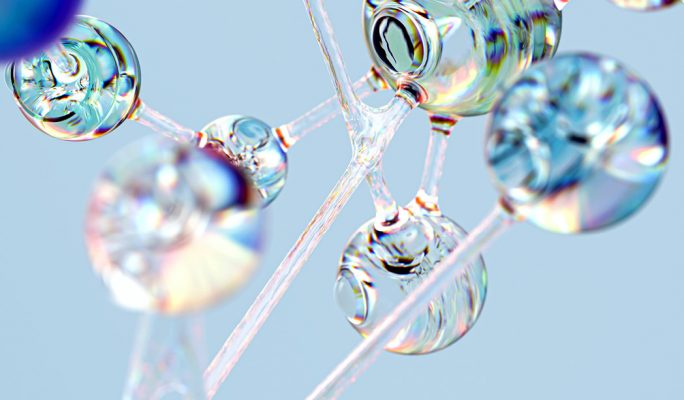
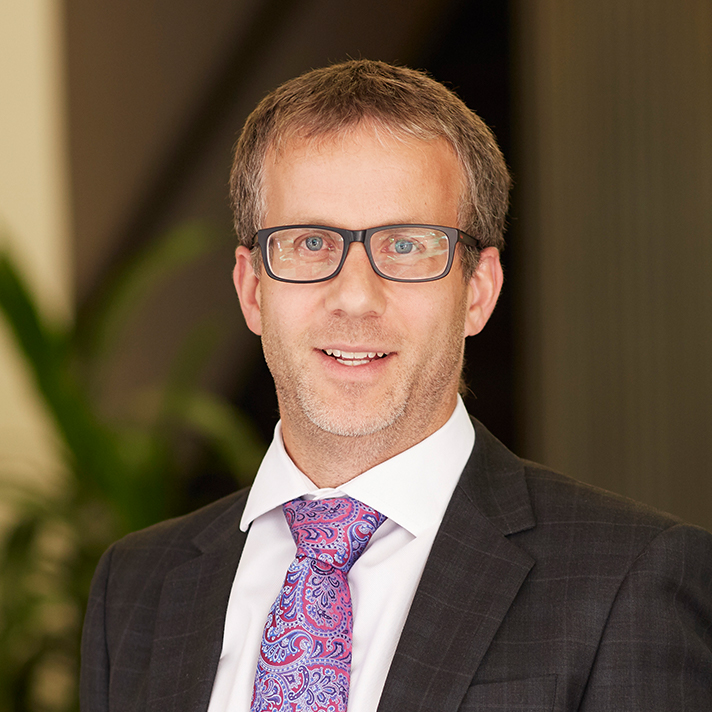
Lessons learned one year on – Algorithm charter for Aotearoa New Zealand
Take a look at the lessons learned from Taylor Fry's newly released review of the Algorithm charter for Aotearoa New Zealand
Read Article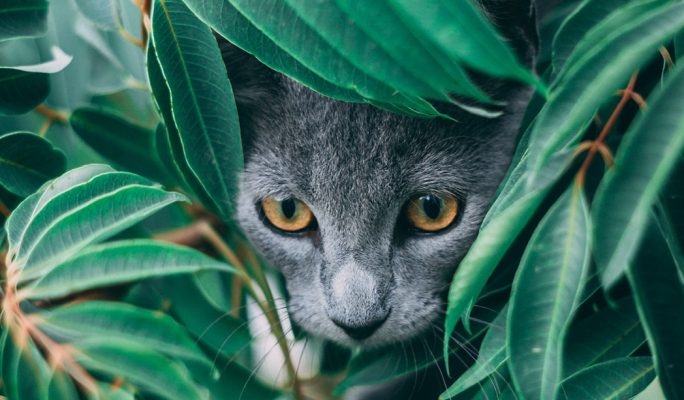
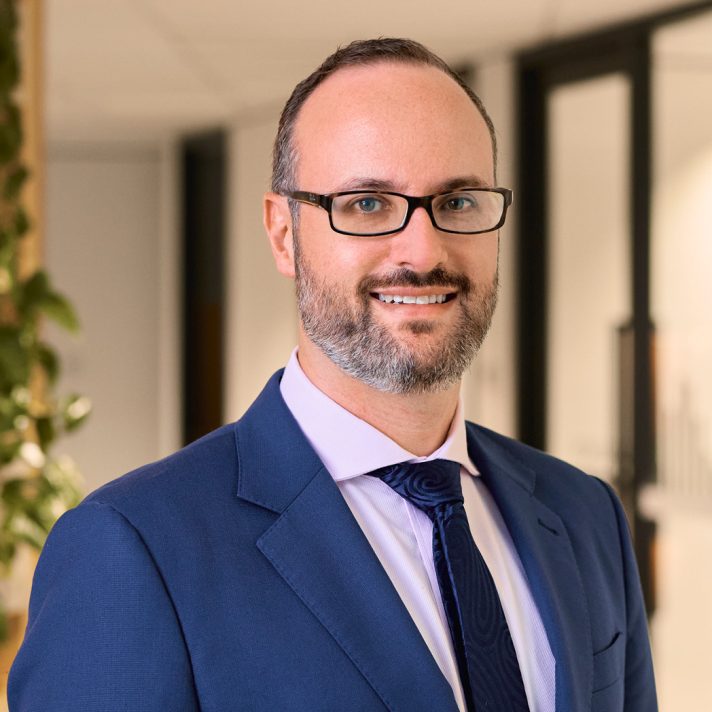
The Australian privacy act is changing – how could this affect your machine learning models?
In the first of a two-part series, we look at the proposed changes, their potential impacts for industry and consumers, and what you can do
Read Article